AI’s GPU obsession blinds us to a cheaper, smarter solution
The post AI’s GPU obsession blinds us to a cheaper, smarter solution appeared on BitcoinEthereumNews.com. Opinion by: Naman Kabra, co-founder and CEO of NodeOps Network Graphics Processing Units (GPUs) have become the default hardware for many AI workloads, especially when training large models. That thinking is everywhere. While it makes sense in some contexts, it’s also created a blind spot that’s holding us back. GPUs have earned their reputation. They’re incredible at crunching massive numbers in parallel, which makes them perfect for training large language models or running high-speed AI inference. That’s why companies like OpenAI, Google, and Meta spend a lot of money building GPU clusters. While GPUs may be preferred for running AI, we cannot forget about Central Processing Units (CPUs), which are still very capable. Forgetting this could be costing us time, money, and opportunity. CPUs aren’t outdated. More people need to realize they can be used for AI tasks. They’re sitting idle in millions of machines worldwide, capable of running a wide range of AI tasks efficiently and affordably, if only we’d give them a chance. Where CPUs shine in AI It’s easy to see how we got here. GPUs are built for parallelism. They can handle massive amounts of data simultaneously, which is excellent for tasks like image recognition or training a chatbot with billions of parameters. CPUs can’t compete in those jobs. AI isn’t just model training. It’s not just high-speed matrix math. Today, AI includes tasks like running smaller models, interpreting data, managing logic chains, making decisions, fetching documents, and responding to questions. These aren’t just “dumb math” problems. They require flexible thinking. They require logic. They require CPUs. While GPUs get all the headlines, CPUs are quietly handling the backbone of many AI workflows, especially when you zoom in on how AI systems actually run in the real world. Recent: ‘Our GPUs are melting’ — OpenAI puts…
The post AI’s GPU obsession blinds us to a cheaper, smarter solution appeared on BitcoinEthereumNews.com.
Opinion by: Naman Kabra, co-founder and CEO of NodeOps Network Graphics Processing Units (GPUs) have become the default hardware for many AI workloads, especially when training large models. That thinking is everywhere. While it makes sense in some contexts, it’s also created a blind spot that’s holding us back. GPUs have earned their reputation. They’re incredible at crunching massive numbers in parallel, which makes them perfect for training large language models or running high-speed AI inference. That’s why companies like OpenAI, Google, and Meta spend a lot of money building GPU clusters. While GPUs may be preferred for running AI, we cannot forget about Central Processing Units (CPUs), which are still very capable. Forgetting this could be costing us time, money, and opportunity. CPUs aren’t outdated. More people need to realize they can be used for AI tasks. They’re sitting idle in millions of machines worldwide, capable of running a wide range of AI tasks efficiently and affordably, if only we’d give them a chance. Where CPUs shine in AI It’s easy to see how we got here. GPUs are built for parallelism. They can handle massive amounts of data simultaneously, which is excellent for tasks like image recognition or training a chatbot with billions of parameters. CPUs can’t compete in those jobs. AI isn’t just model training. It’s not just high-speed matrix math. Today, AI includes tasks like running smaller models, interpreting data, managing logic chains, making decisions, fetching documents, and responding to questions. These aren’t just “dumb math” problems. They require flexible thinking. They require logic. They require CPUs. While GPUs get all the headlines, CPUs are quietly handling the backbone of many AI workflows, especially when you zoom in on how AI systems actually run in the real world. Recent: ‘Our GPUs are melting’ — OpenAI puts…
What's Your Reaction?
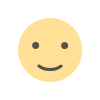
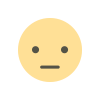
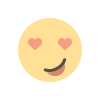
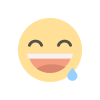
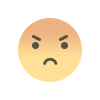
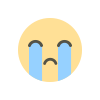
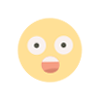