Memoria Unleashes Transformers’ Hidden Power
The post Memoria Unleashes Transformers’ Hidden Power appeared on BitcoinEthereumNews.com. TLDR Hebbian memory elevates transformer models for complex tasks. Memoria’s long-sequence processing empowers transformer performance. Open-source Memoria offers global developers a transformative AI tool. In a groundbreaking development, researchers at Sungkyunkwan University in South Korea have unveiled a novel memory system inspired by the principles of Hebbian theory. This cutting-edge innovation promises to significantly enhance the performance of transformer-based machine learning models, particularly in tasks involving the processing of long data sequences. The research, published on the arXiv preprint server, introduces “Memoria,” a general memory network that leverages Hebbian theory to improve long-term dependencies in neural networks. While transformers have proven to be formidable tools in processing sequential data, they have limitations when it comes to handling longer sequences. Transformers’ restricted storage capacity impedes their ability to effectively process extended input sequences, leading to a decline in performance. Unlike humans, who selectively remember and utilize relevant information from inputs, transformers process all raw data from start to end, making them less efficient in handling lengthy sequences. A Hebbian memory for transformers Researchers Sangjun Park and JinYeong Bak’s primary objective was to design a system that could overcome these limitations and empower transformer models with improved capabilities. Drawing inspiration from Hebbian theory, which posits that neurons and cells that are repeatedly activated together tend to form associations that lead to learning, they introduced Memoria. “Memoria stores and retrieves information called engram at multiple memory levels of working memory, short-term memory, and long-term memory, using connection weights that change according to Hebb’s rule,” explain Park and Bak in their paper. In a series of experiments, the researchers evaluated the effectiveness of their Hebbian memory system, and the results were highly promising. Memoria demonstrated its ability to significantly enhance the performance of transformer models across a range of tasks that involve processing lengthy…

The post Memoria Unleashes Transformers’ Hidden Power appeared on BitcoinEthereumNews.com.
TLDR Hebbian memory elevates transformer models for complex tasks. Memoria’s long-sequence processing empowers transformer performance. Open-source Memoria offers global developers a transformative AI tool. In a groundbreaking development, researchers at Sungkyunkwan University in South Korea have unveiled a novel memory system inspired by the principles of Hebbian theory. This cutting-edge innovation promises to significantly enhance the performance of transformer-based machine learning models, particularly in tasks involving the processing of long data sequences. The research, published on the arXiv preprint server, introduces “Memoria,” a general memory network that leverages Hebbian theory to improve long-term dependencies in neural networks. While transformers have proven to be formidable tools in processing sequential data, they have limitations when it comes to handling longer sequences. Transformers’ restricted storage capacity impedes their ability to effectively process extended input sequences, leading to a decline in performance. Unlike humans, who selectively remember and utilize relevant information from inputs, transformers process all raw data from start to end, making them less efficient in handling lengthy sequences. A Hebbian memory for transformers Researchers Sangjun Park and JinYeong Bak’s primary objective was to design a system that could overcome these limitations and empower transformer models with improved capabilities. Drawing inspiration from Hebbian theory, which posits that neurons and cells that are repeatedly activated together tend to form associations that lead to learning, they introduced Memoria. “Memoria stores and retrieves information called engram at multiple memory levels of working memory, short-term memory, and long-term memory, using connection weights that change according to Hebb’s rule,” explain Park and Bak in their paper. In a series of experiments, the researchers evaluated the effectiveness of their Hebbian memory system, and the results were highly promising. Memoria demonstrated its ability to significantly enhance the performance of transformer models across a range of tasks that involve processing lengthy…
What's Your Reaction?
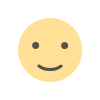
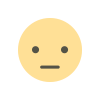
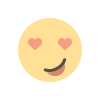
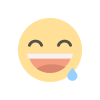
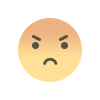
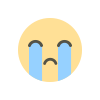
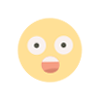